If you’re trying to build out a great data team in your company, you’ve probably noticed that there are lots (and lots and lots) of analytics and data roles out there. And so many of those data job titles sound confusingly similar. it can be hard to make sense of it all, even if you’re in the field.
In this post (1 of 4) we’ll give you a big-picture overview of data roles and responsibilities. We’ll also define the three functional areas: data science, data engineering, and data analytics. In subsequent posts, we’ll dive deeper into each area and explain some of the more common roles within them.
It starts with your data…
Almost every business, large and small, gets data from inside and outside. As a simple example, here at Dataspace we have the following, internal systems. These are separate—each creates its own data:
- Accounting / ERP
- Staff planning and forecasting
- Applicant tracking
- CRM
- Various spreadsheets and documents that get used, updated, and shared every day
But, that isn’t all the data we need to access. There is also external data, such as:
- Google data about our search rankings
- LinkedIn data about our posts and candidates to whom we’re reaching out
- Bank account data about deposits, payments, and scheduled EFTs
- And more…
Suppose I need a comprehensive view of a customer: How did they find us? Which salesperson served them? How much business have they done with us over time? Who were the consultants and recruiters we assigned to work with them? What newsletter topics interested them most?
Now, suppose I need that view over all customers, so I can answer questions such as: What has the trend in sales been for the past three years and what does that look like if I extend it for the next year? Is there a correlation between the number of newsletters we send and the number of customers we have? How long does it take to receive payment after we send an invoice, and what does that imply for our cash flow?
To address either of these situations I could spend lots of time going to each system to get the data I need. But, even then I will need to bring it together from the different systems that hold it. For example, if I want to know if people who read our newsletter spend more or less with us than people who don’t, I need to be able to tie my accounting data to my CRM data.
To make this data accessible to everyone who might need to analyze and report on it, we need a data architecture to connect it. I need something like this:
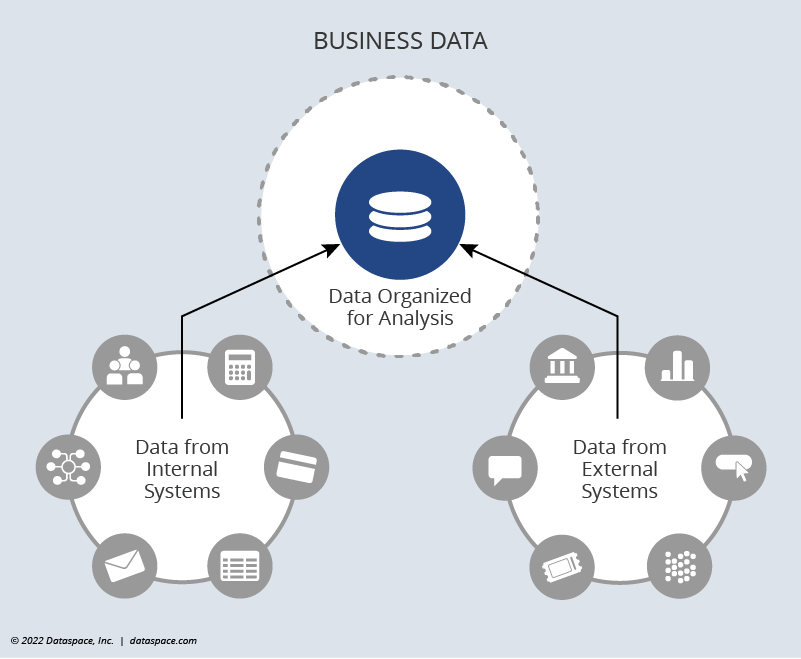
Now we are set up for data analysis! With that background, let’s look at what the three major data roles (data analyst, data scientist, and data engineer) do and how they need to interact with this system and the data within it.
Putting your data to work: the main data roles
At Dataspace, we divide data-focused roles into three major functional areas: data analysts, data engineers, and data scientists. This scheme seems pretty common (although many organizations still do it differently), and it helps us streamline the hiring process for data and analytics positions. But it still can be a bit perplexing, especially when varied data responsibilities get lumped into one role, which is not uncommon in SMBs and startups.
Let’s look at each of these roles and how they interact with the data architecture we just laid out.
Data analysts tell me about my business
Data analysts are, by far, the data roles that are most in demand. In 2020, jobs in analytics made up 67% of data science hires in the U.S. This may seem surprising g due to all the hype data scientists get lately.
So what exactly do data analysts do? What makes their role distinct from data engineers and data scientists? In the broadest sense, a data analyst takes the data collected by a business and uses it to describe what’s going on. They select, combine, and assess that data to identify trends and extract insights. Data analysts frequently present their analyses in reports, dashboards, and visualizations. Business managers use these reports to help them make decisions.
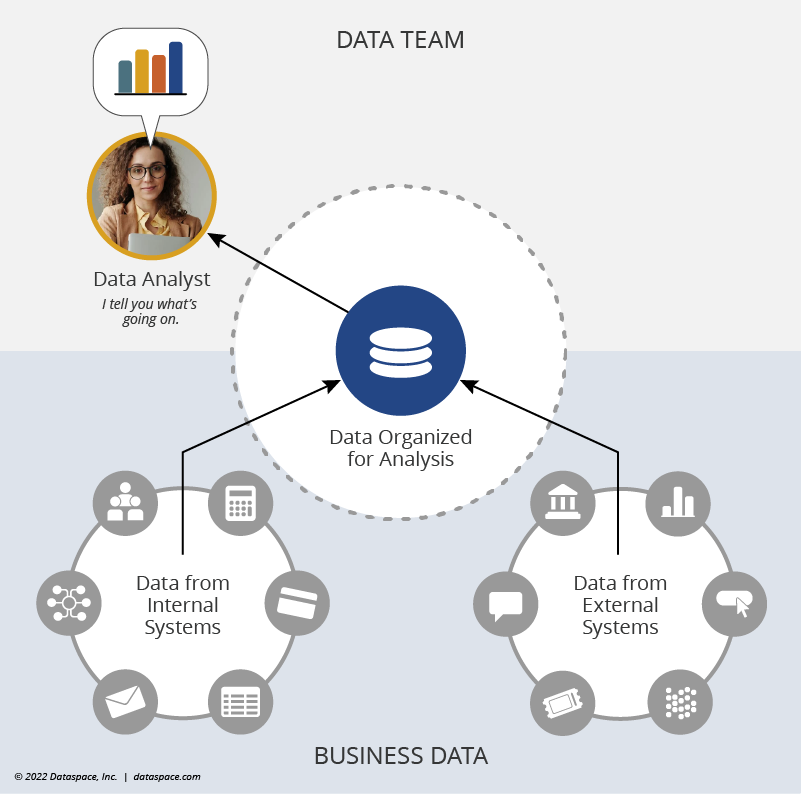
If I want to know the trend in sales for the past four years, I call a data analyst. If I want to see that sales trend plotted next to the trend in the number of people we employ, I call a data analyst. That data analyst is describing my world and telling me about my business.
Data analysts are adept in query and analysis tools, such as SQL and Excel, as well as business intelligence tools, such as Power BI and Tableau.
There are many different subtypes of data analyst roles. Analysts can focus on understanding different types of business data. Examples include, business intelligence analysts, marketing analysts, and product analysts. While other analysts focus on different points in the process of understanding it. This includes roles such as analytics developers, data reporting analysts, and data visualization specialists.
We dive deeper into the role of data analysts in an organization in a later post.
Data scientists predict the future
While the data analyst describes what’s going on in the business now, the data scientist predicts what will happen in the future. The data analyst tells you who responded to particular promotions. The data scientist tells you the likely effect of changes to those promotions.
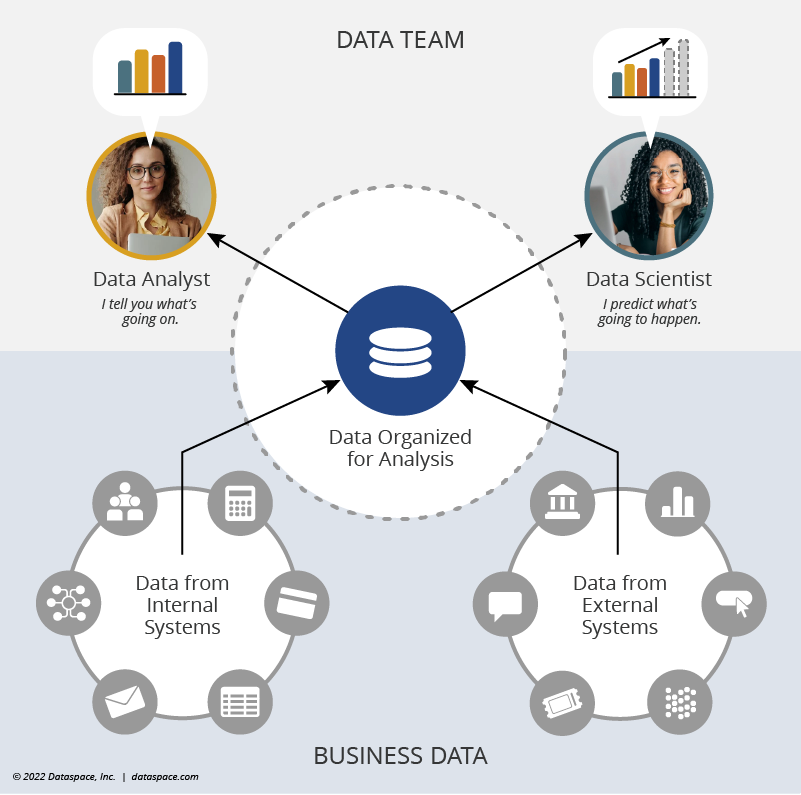
To understand the data role of the data scientist, it’s important to know that some things that don’t seem like predictions are, in actuality, predictions. For example, facial recognition technology is actually a prediction. It’s the result of a data science algorithm that “guesses” whose face it’s seeing, with a certain level of confidence. Facial recognition predicts—with a very high level of confidence—that it’s actually you (hopefully) who’s trying to access your iPhone.
Data scientists use many of the same skills as data analysts, but they must also be able to apply advanced statistical techniques. So, they will typically know some SQL, but also programming languages like Python, as well as data-science specific languages like R and SAS.
The difference between data analysts and data scientists is easy to confuse. It may be tempting to think of the data analyst as a junior role to the data scientist, yet the reality is not so simple. Both data roles will determine the data they need, decide how to combine it, and then analyze it in a variety of ways. But there’s a big difference in how they seek to understand the data they work with. The article, Distinguishing Data Roles: Engineers, Analysts, and Scientists, (which offers a great primer on data science roles) sums it up nicely:
“A data scientist should be able to sift through data in the same way as an analyst, but also be able to apply statistical techniques in order to differentiate between signal and noise.”
Data scientists tend to command higher salaries than data analysts, due to the advanced skills required for the role. Employers frequently require that data scientists have advanced degrees. (It’s not unusual for Physics PhDs to make excellent data scientists.) As such, they are more likely to be employed by enterprise organizations. As a sort of “elite” role, data scientists made up only about 10% of the data-focused hires in 2020.
Of the three major data science functions we describe in this post, data scientist appears to have least variability in job titles. (Of all the data science roles we worked on in 2021, only 2 did not have “data scientist” in the title.) We take a more in depth look at the value data scientists provide an organization in a future post.
Data engineers build the systems that data analysts and data scientists need
In contrast to data scientists and analysts who ‘suck data out of’ the data pipeline, data engineers work on the other end of that pipeline—they are the ones who build and maintain it. As a whole, data engineers ensure raw data is accurately collected, securely and reliably stored, and structured for easy use by data scientists and analysts.
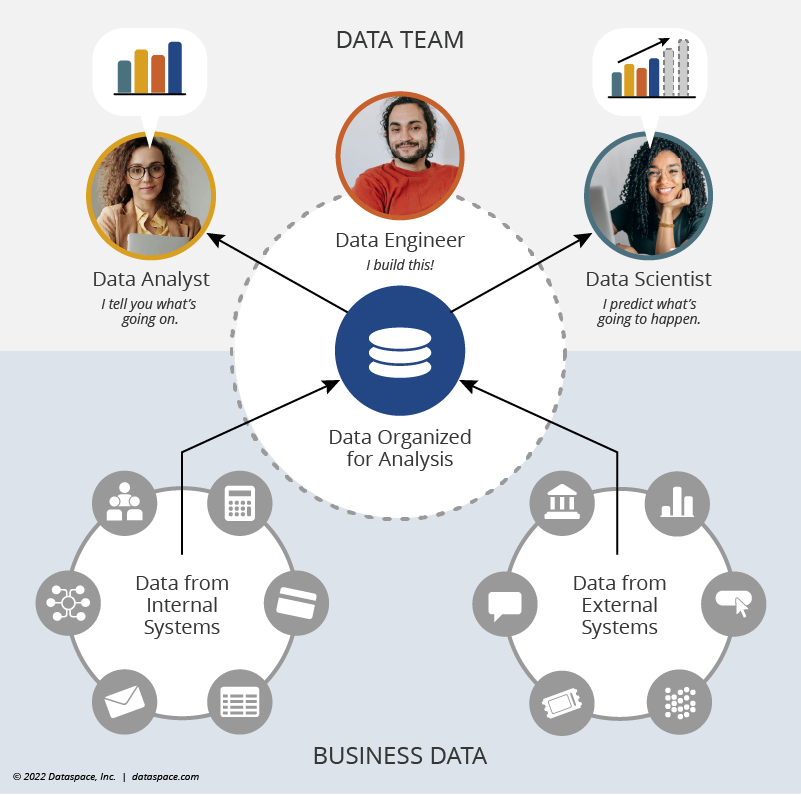
Within the role of data engineering, there are different sub-roles at each phase of that process. There are developers who focus on specific languages or technologies, cloud and warehouse architects, and ETL and data transformation specialists. All of these data engineers require strong technical skills, such as programming and cloud management. Data engineers are vital members of a mature data team. It often takes many data engineers with varying specialties to build and manage the pipelines needed by a single data scientist. As such a high-value data role, data engineers made up about 23% of all data hires in the U.S. in 2020, with salaries that rival, or even exceed, data scientists.
Data engineers use a variety of data movement and integration technologies. They are usually highly skilled in SQL. They also frequently know cloud technologies such as AWS, Azure, and GCP; ETL tools such as Informatica and SSIS; and scripting and programming languages such as Python and Linux shell scripting.
It’s hard to imagine that data engineers won’t continue to be highly valuable in the decades to come. While the demand for specific skills will shift, (for example, the demand for cloud data engineering roles now vastly outpacing traditional on-prem ones), businesses will continue to need data engineers who can adapt to and implement new technologies
It’s important to note; you don’t always need a data engineer on your data team. Data engineers are often employed by larger companies or organizations that work with large data sets from multiple sources. Remember that most data engineer roles exist to support data analysts and data scientists. However, data analysts and data scientists already have some level of technical competence. Many organizations can get the data pipelines they need without employing data engineers. In fact, it’s not unusual to see organizations start their analytics journey by hiring a data analyst. Over time, as the analytic needs grow, they add more analysts. Eventually, they may add data scientists and data engineers, often at the same time.
We will break down the roles of data engineers in supporting business analytics in a later post.
Need help filling data roles on your team?
We are experts at finding and vetting highly-qualified data scientists, data engineers, and data analysts, because we are “data people,” too. Contact us to get started.